AI is no longer a luxury; it's a necessity. Yet, many businesses struggle to keep up. The fear of be...
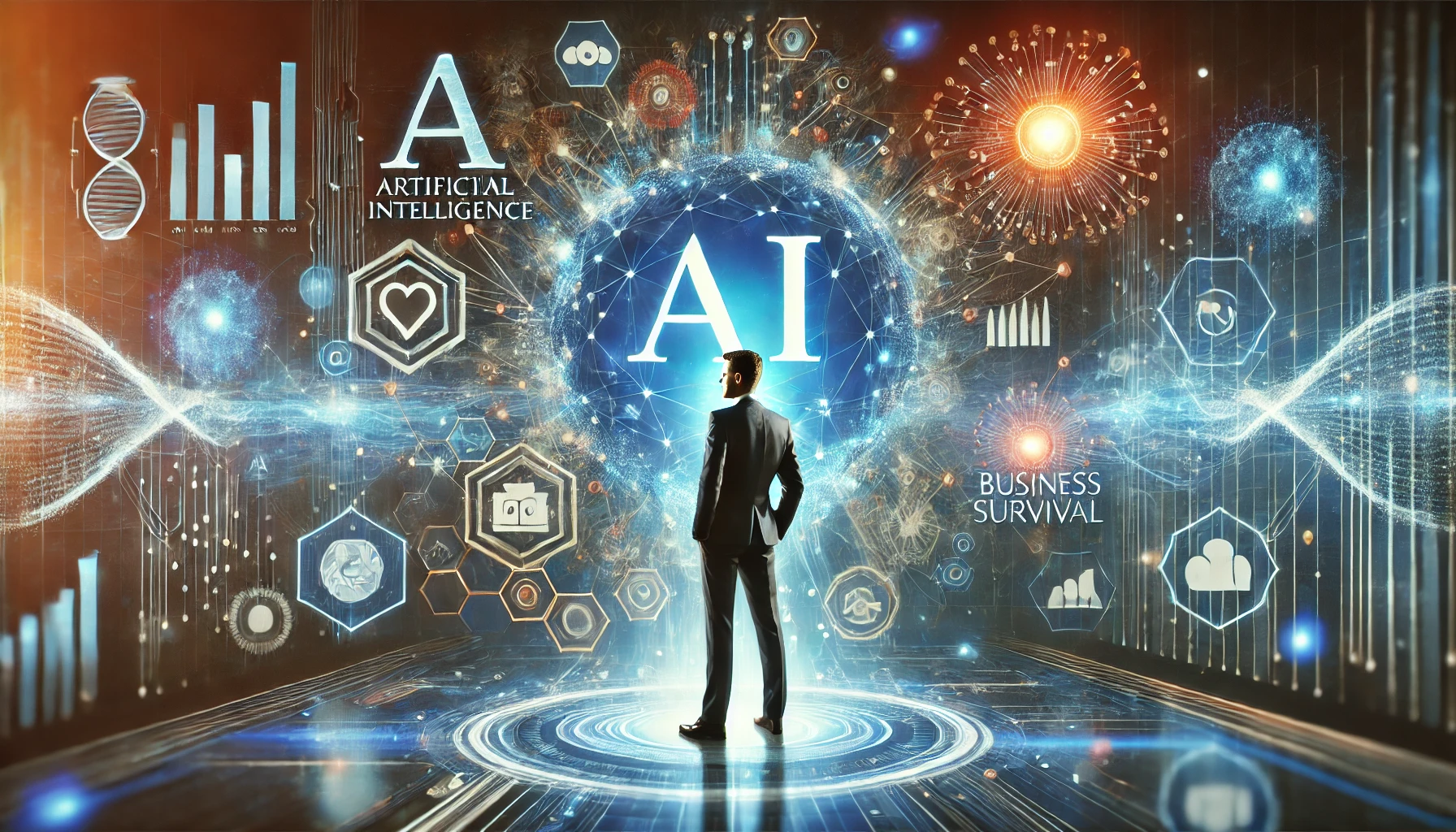
Explore insightful articles on Natural Language Processing, Artificial Intelligence, and software development trends, featuring tutorials, analyses, and innovative perspectives.
AI is no longer a luxury; it's a necessity. Yet, many businesses struggle to keep up. The fear of be...
In a digital age that moves at lightning speed, it's easy to feel overwhelmed by the endless stream ...
A tutorial with code using PySpark, Hugging Face, and AWS GPU instances Are you looking for up to a ...
The horror of horrors! I am that ancient in the land of software development. I have been messing ar...
I have implemented several predictive models using Random Forests. Here are my thoughts on why they ...
Here are some thoughts on Cathal Horan's article "When Not to Choose the Best NLP Model." The world ...
Being creative or artistic has long been the sole domain of humans. What if machines can be as creat...
What is the underlying focus of a KPI? It could be a telltale sign of the organization’s culture. An...
How about digital blackboards? I will try digital blackboard presentations to see how that goes, mai...
Twenty years after Deep Blue defeated the World Champion at Chess, Alpha Go did the same for the Wor...
If you found our insights on Natural Language Processing, Artificial Intelligence, and software development trends valuable, imagine what you could achieve with our expertly crafted products and services. Whether you’re looking to integrate cutting-edge technology into your projects, streamline your development process, or stay ahead of the latest industry innovations, we have the tools and expertise to support you every step.
Contact us to learn more about our offerings and discover how our tailored solutions can empower your business to innovate, grow, and succeed.